BACKGROUND
Certain characteristics of online communications, such as anonymity, accessibility and reproducibility, create conditions conducive to aggressive actions (Tokunaga, 2010). One form of such actions, cyber-bullying, has recently attracted attention because of its increasing occurrence and serious consequences for victims (Park et al., 2021; Zhu et al., 2021). Nearly all young people have access to the internet, and, as mentioned in a study from a couple of years ago, adolescents usually spend 17 to 40 hours per week online (Kowalski et al., 2014). On one hand, this gives some of them an ideal opportunity to express their aggression; however, it makes many other internet users vulnerable to such actions. Research on cyber-bullying usually concentrates on the characteristics of perpetrators, the predictors of this specific form of aggression, as well as on the victims in terms of the individual and social negative consequences. There is strong agreement among researchers that cyberbul-lying is an aggressive and pathological form of communication that should be eliminated and prevented by all means (Park et al., 2021; Tokunaga, 2010; Zhu et al., 2021).
CYBERBULLYING – DEFINITION
Definitions of cyberbullying usually treat it as a specific form of aggressive behaviour which involves the use of electronic media to bully others. According to Tokunaga (2010), it is any behaviour performed through electronic media that communicates hostile or aggressive messages intended to inflict harm or discomfort on others. Kowalski et al. (2012) defined cyberbullying as aggression that is intentionally and repeatedly carried out in an electronic context against a person who cannot easily defend him- or herself. Patchin and Hinduja (2006) described cyber-bullying as the wilful use of information and communication technology as a medium through which harm or discomfort is intentionally and repeatedly inflicted on a specific person or group of persons. Their definition was expanded later by adding the element of imbalance of power as a key characteristic of cyberbullying (Patchin & Hinduja, 2016).
While some researchers define cyberbullying simply as a form of bullying that occurs via electronic media, others develop the concept by stating that there are different forms of such behaviours. Willard (2007) proposed a typology of cyberbullying forms which includes: flaming (i.e., an online fight), harassment (i.e., repetitive, offensive messages sent to a target), outing and trickery (i.e., soliciting personal information from someone and then electronically sharing that information with others without the individual’s consent), exclusion (i.e., blocking an individual from buddy lists), impersonation (i.e., posing as the victim and electronically communicating negative or inappropriate information with others as if it were coming from the victim), cyber-stalking (i.e., using electronic communication to stalk another person by sending repetitive threatening communications), and denigration (i.e., slander with text messages or emails; posting defamatory comments or false rumours).
On the conceptual level, there is a question concerning the differences between traditional bullying and cyberbullying. According to Donegan (2012), traditional bullying evolved into a form called cyberbul-lying. Both forms are of a similar nature, but there are also some important differences. Perpetrators of cyberbullying usually, but not always, perceive themselves to be anonymous (Kowalski et al., 2014). Moreover, there are some features of cyberspace, such as not being in the same room and the filtering role of the computer screen, which can hide or distort some reactions of the victims. While this may encourage cyberbullies to take such actions, there is also an adverse effect: they cannot observe the impact on the victim and thus chances for empathy are reduced. Moreover, victims of cyberbullying are accessible at any time, but traditional bullying is limited to time spent at school premises or school-related activities. Additionally, it should be mentioned that there is a much broader audience on the Internet, which has as a medium unlimited capacity, and on the other hand bystanders can take many roles. Considering those differences, a hypothesis was formulated that perpetrators have different motives for the two types of bullying behaviour: more intrapersonal in the case of cyberbullying; and more interpersonal in the case of traditional bullying (Kowalski et al., 2014). Intra- personal motives in the case of cyberbullying serve as means of regulating negative mood. Similar factors, such as anonymity and accessibility, were also mentioned by Tokunaga (2010), who also indicated another factor, lack of supervision in electronic media in case of cyberbullying while instructors and school administrators serve as agents of enforcement in traditional bullying. Moreover, three necessary conditions have to be met in the case of cyberbullying: the behaviours must be repeated, involve psychological torment, and be carried out with intent (Tokunaga, 2010). On the other hand, as pointed out by Tokunaga (2010), bullying and cyberbullying share considerable overlap in their core motivations – the intention to inflict harm on others.
PREVALENCE OF CYBERBULLYING
Electronic media have recently become a new and rapidly developing arena for social interactions. Probably as a consequence of this, the occurrence of cyberbullying doubled between 2007 and 2016, and the percentage of individuals who have experienced cyberbullying at some point in their life is reported to be between 18% and 34% (Patchin & Hinduja, 2016). A systematic review of studies on cyberbullying in East Asia (Park et al., 2021) indicated that the frequency of reported experience of cyberbullying ranged from 5.4% to 56.8% in different nations. Another analysis, based on 63 studies (Zhu et al., 2021), showed prevalence rates between 14.6% and 52.2% for bullying victimization and 6.3% to 32% for cyberbul-lying perpetration. According to Atkins et al. (2020), 39% of students reported experiencing cyberbullying. Kowalski et al. (2014) found that 15% to 18% of adolescents had been cyberbullied, while the percentage of perpetrators ranged between 7 and 18. Other studies generally estimate that cyberbullying is reported by 10 to 40 percent of respondents (Lenhart et al., 2010). As suggested by Kowalski et al. (2014), such discrepancies may by partially attributed to different criteria of classification of bullying behaviours.
CONSEQUENCES OF CYBERBULLYING
While it could be expected that the negative consequences of cyberbullying concern victims, studies show that both victims and perpetrators show symptoms of depression and anxiety (Chang et al., 2013; Didden et al., 2009), substance abuse and somatic dysfunctions (Kowalski & Limber, 2013), decreased self-esteem (Didden et al., 2009), suicidal ideation (Hinduja & Patchin, 2010), increased likelihood of self-injury (Schneider et al., 2012) and loneliness (Sahin, 2012). Both groups show impaired performance at school (Holfeld & Grabe, 2012), and they are more likely to be absent from school and receive low grades (Li, 2005). Moreover, negative changes have been observed, such as changing schools or repeating the same grade. It was also reported that cyber-bullying victims showed impaired social functioning (Gündüz et al., 2021).
RISK FACTORS OF CYBERBULLYING
Studies show that certain individual characteristics make a person more inclined to engage in cyberbul-lying or more prone to experiencing such actions. For instance, lower levels of cognitive empathy (under-standing other people’s emotional states) and affective empathy (affectively responding to other people’s emotional states) were related to cyberbullying independently of the measures used (Ang & Goh, 2010; Schultze-Krumbholz & Scheithauer, 2009; Steffgen et al., 2011). It was also found that victimization was negatively associated with cognitive empathy but not with affective empathy. A review of empirical studies showed that lower empathy was generally associated with direct bullying behaviour but not necessarily with the use of electronic media (Dodaj et al., 2013). Perpetrators were also found to be more narcissistic (Ang et al., 2011) and had high levels of anger (Gradinger et al., 2012). When bullying behaviours in general were considered, it was found that perpetrators scored higher on assertiveness, while victims scored lower (Ireland, 2010). Hyperactivity and low social intelligence were also found to be related to victimization (Dooley et al., 2010; Hunt et al., 2012).
Cyberbullies have been found to have been exposed to more environmental violence and exhibit a tendency towards rule-breaking behaviours (Ybarra & Mitchell, 2004). They have also been found to have lower school commitment, they dislike school more (Kowalski & Limber, 2013), they have poor relationships with their parents, and they are mainly brought up by authoritarian parents with a lack of parental attention, love and acceptance (Dilmac & Aydoğan, 2010).
Another risk factor is related to values and perceptions. Moral approval of cyberbullying and moral disengagement was found to make an individual more likely to be a perpetrator (Pornari & Wood, 2010; Walrave & Heirman, 2011; Williams & Guerra, 2007). Generally, perpetrators tend to perceive their actions as less harmful and more benign in intent; they also show a tendency to morally disengage.
Research results seem to be rather inconclusive in the case of gender differences. Numerous studies have shown that boys are more likely to become perpetrators (Dehue et al., 2008; Olweus, 2010), while girls are more often victims (Smith et al., 2008). Other studies have reported no significant difference (Hinduja & Patchin, 2008; Mishna et al., 2010; Slonje & Smith, 2008) or have found girls to be more involved in cyberbullying than boys (Kowalski & Limber, 2007).
PROTECTIVE FACTORS OF CYBERBULLYING
Most of the individual characteristics, motives and perceptions that have been discussed as risk factors can serve as protective factors when they have the opposite character; for example, being more empathic prevents an individual from engaging in cyberbullying. Two specific significant protective factors have been reported, namely social support and prosocial attitudes. Cyberbullying in general was found to be associated with poor social support (Fanti et al., 2012). Adolescents who had good relations with peers and perceived more social support from their friends were less likely to be victims (Calvete et al., 2010). They were also less prone to victimization when they felt less lonely (Sahin, 2012) and not socially isolated (Siegle, 2010). Those who reported more parental control and support as well as stronger emotional bonds with their parents were less likely to be perpetrators (Hinduja & Patchin, 2013; Ybarra & Mitchell, 2004). Moreover, Williams and Guerra (2007) also found that less peer support predicted all types of bullying, including internet bullying. On the other hand, a positive school environment that is perceived as being trusting, fair and pleasant was related to less bullying (Williams & Guerra, 2007).
In the case of bullying victimization, a negative relationship was identified between parental control of technology and cybervictimization (Aoyama et al., 2012). Victims have also been found to have more problematic relations with their parents (Accordino & Accordino, 2011; Makri-Botsari & Karagianni, 2014).
The protective value of prosocial attitudes is less documented in the literature, but it is expected to make adolescents less inclined to engage in cyberbul-lying (Kowalski et al., 2014). It has also been suggested that a feeling of happiness and prosocial behaviour mutually reinforce each other through a positive feedback loop (Erreygers et al., 2018). Other studies with samples from all ages indicated that offline prosocial behaviour and happiness are related (Aknin et al., 2013, 2015; Nelson et al., 2016; Otake et al., 2006).
Most of the results mentioned above were based on cross-sectional research, so the direction of causality could not be determined definitely. However, considering the relatively stable nature of some of the risk/protective factors, we may expect such causality, as in the case of cognitive empathy, hyperactivity or social intelligence.
CURRENT STUDY
Providing empirical data concerning the prevalence of cyberbullying as well as identifying the factors that make a person more or less inclined to cyber-bullying or being more vulnerable to victimization could help in developing prevention programmes at schools and make teachers and parents more aware of the problem.
The study reported here had two major purposes. Firstly we wanted to determine the prevalence rates of cyberbullying in Polish adolescents, considering the fact that there were great discrepancies in rates reported in studies of other nations (Atkins et al., 2020; Park et al., 2021). In order to cover the whole context of cyberbullying, we decided to include four types of respondents: bullies only; victims only; bully-victims; no bullies and no victims.
Secondly, the study aimed to examine the association between cyberbullying and factors such as cognitive empathy, assertiveness, cooperation, pro-social behaviour, and social support (family, peer and teacher) which may serve as protective or risk factors of cyberbullying. The protective or risk nature of those factors depends on their level, i.e. low social support could serve as a risk factor, high social support as a protective factor.
PARTICIPANTS AND PROCEDURE
PARTICIPANTS
Participants were 822 students who were recruited from grades 6 to 8 of Polish public primary schools (grade 6 = 30.2%; grade 7 = 34.3%; grade 8 = 35.5%). The students included 54.5% girls (n = 448) and 45.5% boys (n = 374). The average age was M = 12.97, SD = 1.02.
MEASURES
Disorders in Media-related Functioning Questionnaire. Cyberbullying was measured with 14 items (Likert type scale) from the Disorders in Media-related Functioning Questionnaire (DMRF-Q; in Polish – KFS-PM-C; Wysocka et al., 2021), which covers different forms of cyberbullying: flaming, harassment, cyberstalking, denigration, impersonation, outing and trickery, exclusion. There are 7 items that concern engaging in cyberbullying behaviours (for example, “In the last 6 months, how many times have you sent someone a text message or instant message in order to mock them?”); 7 items concern experiencing cyberbullying (for example, “In the last 6 months, how many times have you received threats over the internet or on a mobile phone that caused you to be concerned about your safety?”). Both scales have very good psychometric properties. Engaging in cyberbul-lying: model fit – RMSA = .039, CFI = .990, TLI = .986; reliability – Cronbach’s α = .91, ω = .92. Experiencing cyberbullying: model fit – RMSA = .028, CFI = .995, TLI = .993; reliability – Cronbach’s α = .90, ω = .91 (Wysocka et al., 2021).
Protective factors were subscales of the two questionnaires: social support peer (SSP); social support teacher (SST); social support family (SSF); pro-social behaviour (PSB); assertiveness (AS); cognitive empathy (CE) and cooperation (CO). All items were Likert type scales.
Social Relationship Functioning Questionnaire (SRF-Q; in Polish: KFS-S-RS by Wysocka et al., 2021) for the assessment of resources, skills, abilities or competences related to the perception of the functioning in interpersonal relations, such as empathic abilities, assertive abilities, cooperative abilities, and pro-social behaviour (model fit: RMSA = .054, CFI = .995, TLI = .946; reliability: Cronbach’s α .73 to .81) (Wysocka et al., 2021).
The Educational Relationship Functioning Questionnaire (ERF-Q; in Polish: KFS-S-RE by Wysocka et al., 2021) measures individual characteristics that are important in the process of learning and coping in school situations, where it is important to perceive support that is potentially available in the social environment, such as support from the peer group, support from teachers and school staff, and support from parents and guardians (model fit: RMSEA = .041, CFI = .970, TLI = .966; reliability: Cronbach’s α .76 to .89) (Wysocka et al., 2021).
PROCEDURE
The research covered the whole of Poland (16 provinces). The participants were recruited from 55 grades of 26 primary schools. Three types of locality were selected – big cities (over 200,000 residents), cities (up to 199,000 residents) and villages (up to 10,000 residents). Schools were randomly selected separately in three types of locality and then grades from those schools were randomly selected as well. School authorities were then contacted and asked for contact with parents. Parents from selected grades were then asked to accept their children’s participation in research. Written parental consent was required. Then students were asked for consent.
Data were collected at the end of October 2019 and the beginning of March 2020 using the computer-assisted self-interview (CASI), which was applied in the form of a computer-based online questionnaire that was completed by each student (in the presence of a trained interviewer/instructor). Data collection took place during school hours. It took participants 20 to 30 minutes to complete the survey.
Implementation of the research did not require permission to be obtained from an ethics committee – according to the Polish practice (special, separate document). The National Centre for Science and Development (NCBR), while subsidizing the project, obliges the contractors to act in accordance with common ethical standards. The NCBR does not fund unethical research. In addition, the project employed psychologists, pedagogists, educators, and sociologists as expert judges who evaluated each item of the tool in terms of the ethicality of giving it to students. There were no objections to any of the items or the research procedure.
RESULTS
ANALYSIS PLAN
The first phase of the analysis will focus on determining the frequency of the seven forms of cyber-bullying: cyberstalking, denigration, exclusion, flaming, harassment, impersonation, outing and trickery. The frequency with which students experience and use a given form of cyberbullying will be analysed. This phase will be completed by the classification of subjects into one of the four groups: (1) neither experiencing cyberviolence nor using it against peers (no victim and no perpetrator); (2) experiencing cyberbullying but not using it (victim only); (3) both experiencing cyberbullying and applying it to others (victim and perpetrator); (4) using cyberbullying but not experiencing it from others (perpetrator only).
This classification is based on two dichotomous indices: (1) victim or not victim, (2) perpetrator or not perpetrator. The first dichotomous index (victim or not victim) was constructed based on the seven specific questions about the various forms of cyberbullying (flaming, harassment, cyberstalking, denigration, impersonation, outing and trickery, exclusion). To be denoted a victim on the specific measure, a student had to have been bullied at least three times (in the last six months) with at least one of the seven different forms of cyberbullying specified in the questionnaire. We chose the cut-off point of at least three times (in the last six months) in line with: (1) the definition of cyberbullying widely accepted among international researchers (e.g., Hinduja & Patchin, 2008; Olweus & Limber, 2018; Qudah et al., 2019; Smith et al., 2008; Tokunaga, 2010), according to which cyberbullying is not a one-time or occasional event, but a phenomenon repeated over time, and (2) with previous research practice (e.g., Baldry et al., 2016; Kowalski et al., 2012; Olweus, 2012; Solberg & Olweus, 2003).
Consequently, students who had been bullied in the last six months 3 times or more often were classified as victims, and students who had never been victims of cyberbullying in the last six months or had been victims of it once or twice during this period were categorized as non-victims. In the same way we constructed the measure of bullying other students (categorizing a student as a perpetrator or not a perpetrator), defining a perpetrator by engagement in one or more of the seven victimisation behaviours at least three times within the last six months. Combining these two dichotomous measures, we obtained four groups of students: (1) those who were neither victims nor bullies (No Victim–No Perpetrator), (2) those who were victims only (Victim Only), (3) those who were both victims and bullies (Victim– Perpetrator), and those who were bullies only (Perpetrator Only). Note that the procedure of creating four categories of respondents based on two dichotomous variables (those who bully and those who are bullied) is commonly used in research on cyberbullying (e.g., Solberg & Olweus, 2003).
Next, multinomial logistic regression (MLR) analysis (Hosmer & Lemeshow, 2000) was used to explore relevant factors that have an effect on the probability of membership of the three groups, with Non-victim and Non-perpetrator as the reference: Only Victim, Victim and Perpetrator, and Only Perpetrator. As in binary logistic regression, multinomial logistic regression uses maximum likelihood estimation to evaluate the probability of categorical membership. Unlike multiple logistic regression analysis, MLR does not assume that the dependent variable is dichotomous. The outcome can have more than two categories and may be a nominal variable. As in MLR, the dependent variable is represented by multiple binary indicator variables; therefore the multinomial logistic regression estimates a separate binary logistic regression model for each category of dependent variables. Each one gives the effect of the predictors on the probability of success in that category, in comparison to the reference category.
Nine variables were included as predictors in the regression model (gender, age, pro-social behaviour, assertiveness, cognitive empathy, cooperation, social support from peer, social support from teacher, and social support from family). The overall fit of a model was assessed using the likelihood ratio test and the chi-square goodness-of-fit test. The overall goodness of fit was tested using the deviance of the likelihood ratio and the Pearson chi-square test. All analyses were performed using IBM SPSS version 27. All p-values are reported as 2-sided, and statistical significance was defined as p < .05.
EXPERIENCE OF CYBERBULLYING
Analysis of pupils’ responses indicates that cyber-bullying is not common or frequently reported. In the case of all the analysed forms of cyberbullying, the majority of respondents stated that they had not been a victim of cyberbullying in the last six months (see Table 1 and Figure 1). Moreover, there were reasonable differences in the reported frequencies of different forms of cyberbullying.
Table 1
Distribution of respondents according to experienced forms of cyberbullying (Victim) (N = 822)
Figure 1
Distribution of respondents according to regularly experienced (at least three times in the last six months) forms of cyberbullying (Victim) (N = 822)
Note. Yes = Regular (at least three times in the last six months) experience of cyberbulling.
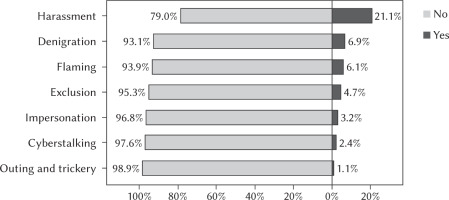
For all seven forms of experience of cyberbullying, a one-sample t-test was performed to determine whether the given form differed from the overall mean (that is from the mean calculated from all forms). Note that because for each form the score could range from 1 to 5, also the overall mean could vary between 1 and 5. In fact, the overall mean was 1.44 (SD = 0.61).
A one-sample t-test showed that the harassment had a significantly (t(821) = 12.81, p < .001, d = .45) higher score than the overall mean (MH = 2.10 vs. MOM = 1.44). The analyses thus indicate that harassment was the most frequently experienced form of cyberbullying: 14.8% of respondents reported experiencing this form four times or more in the last six months, while only 55.2% reported no such experience during that time. In contrast, outing and trickery (M = 1.08, t(821) = –22.74, p < .001, d = –.79), impersonation (M = 1.25, t(821) = –7.42, p < .001, d = –.26) and cyberstalking (M = 1.26, t(821) = –7.24, p < .001, d = –.25) were experienced less often than the overall mean. In the case of these three forms, the percentages of “never” responses were relatively high (correspondingly: 95.3%, 86.1% and 84.4%). Means for the three remaining forms of experience of cyberbullying were not statistically significantly different from the overall score (mean calculated from all forms): for denigration M was 1.50, t(821) = –1.76, p = .081, d = .06); for exclusion M = 1.39, t(821) = –1.62, p = .105, d = –.06; for flaming M = 1.49, t(821) = 1.38, p = .172, d = .05.
As could be expected, pupils reported engaging in different forms of cyberbullying less frequently than experiencing them (see Table 2). The overall score (mean for all forms) was 1.24 (SD = 0.49) and this score was significantly (t(821) = 11.38, p < .001, d = .40) lower than the score for experience of cyber-bullying (M = 1.44, SD = 0.61). In this case, harassment (M = 1.42) and exclusion (M = 1.42) were the most frequently practised forms. For harassment: t(821) = 5.35, p < .001, d = .19; for exclusion: t(821) = 5.44, p < .001, d = .19. Both for harassment and exclusion 3.8% of respondents reported engaging in this form at least four times in the last 6 months. In contrast, the mean scores for cyberstalking (M = 1.14, t(821) = –5.22, p < .001, d = –.18), denigration (M = 1.14, t(821) = –4.88, p < .001, d = –.18), impersonation (M = 1.13, t(821) = –5.92, p < .001, d = –.21) and outing and trickery (M = 1.18, t(821) = –2.72, p < .001, d = –.10) were lower than the overall score (mean calculated from all forms). Only the score for flaming was not statistically significantly different from the overall mean (M = 1.26, t(821) = 0.77, p = .450, d = .03).
Table 2
Distribution of respondents according to use of forms of cyberbullying (Perpetrator) (N = 822)
Both of the aforementioned forms of cyberbul-lying were also most frequently reported to be performed regularly, i.e. at least three times in the last six months: harassment – 5.6%; exclusion – 5.1%. See Figure 2.
Figure 2
Distribution of respondents according to regularly (at least three times in the last six months) use of forms of cyberbullying (Perpetrator) (N = 822)
Note. Yes = Regular (at least three times in the last six months) use of cyberbulling.
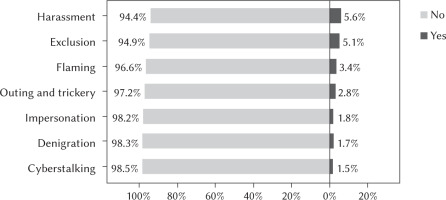
Assuming that being a victim of cyberbullying means experiencing it regularly, respondents were classified as victims (experiencing at least one form of cyberbullying three or more times in the last six months) and not victims (not experiencing any form of cyberbullying three or more times). The analyses shown in Figure 3 panel A indicate that 28.1% of respondents experienced at least one of these forms regularly.
An analogous analysis was performed for engaging in cyberbullying. It was assumed that regularly engaging in such behaviour (perpetrator) means doing at least one of these forms three or more times in the last 6 months. 12.3% of respondents were defined as perpetrators, which was less than half as many persons who experienced cyberbullying (see Figure 3, panel B).
The results of the studies conducted so far indicate that being a victim of cyberbullying does not preclude a person also engaging in such behaviours at the same time. Our analyses (see Figure 3, panel C) show that 68.1% of respondents neither experienced cyberbullying nor engaged in such actions (no victim and no perpetrator). Almost one fifth of pupils (19.6%) experienced cyberbullying but did not perpetrate it (victim only). Almost one in ten (8.5%) respondents both experienced and engaged in cyberbullying (victim and perpetrator). Respondents who performed cyberbullying but did not experience it (perpetrator only) were a relatively small group (3.8%).
CYBERBULLYING – RISK AND PROTECTIVE FACTORS
Descriptive statistics and Pearson correlations for the instruments assessing the cyberbullying risk and protective factors are shown in Table 3.
Table 3
Means, standard deviations, and correlations with confidence intervals
[i] Note. Values in square brackets indicate the 95% confidence interval for each correlation. PSB – pro-social behaviour, Cronbach’s α = .81; AS – assertiveness, Cronbach’s α = .73; CE – cognitive empathy, Cronbach’s α = .74; CO – cooperation, Cronbach’s α = .75; SSP – social support from peer, Cronbach’s α = .85; SST – social support from teacher, Cronbach’s α = .85; SSF – social support from family, Cronbach’s α = .89. *p < .05, **p < .01.
Almost all correlations proved to be statistically significant at the .01 level of confidence. Only the relationship between: (1) assertiveness (AS) and pro-social behaviour (PSB), (2) assertiveness (AS) and social support from teacher (SST) and (3) assertiveness (AS) and social support from family (SSF) are statistically insignificant.
MULTINOMIAL LOGISTIC REGRESSION MODEL
First, a diagnostic examination was conducted on the multinomial logistic regression model with nine predictor variables (gender, age, pro-social behaviour, assertiveness, cognitive empathy, cooperation, social support from peer, social support from teacher and social support from family).
In Table 4 the –2 log-likelihood of the model with only the intercept is 1502.90 while the –2 log-likelihood of the model with the intercept and independent variables is 1381.48. That is the difference (chi-square statistic) is 121.43, which is significant at .05. The null hypothesis that there was no difference between the model without independent variables and the model with independent variables was rejected.
Table 4
Model fitting information with nine predictor variables
Model | Model fitting criterion –2 log likelihood | Likelihood ratio test | ||
---|---|---|---|---|
χ2 | df | p | ||
Intercept only | 1502.90 | |||
Final | 1381.48 | 121.43 | 27 | < .001 |
The Pearson and deviance goodness of-fit statistics are used to test whether the data adequately fit the model. These statistics test the null hypothesis that the model adequately fits the data. If the null is true (the model does adequately fit the data), the Pearson and deviance p-value is small (i.e., less than .05). Our significant values with respect to the Pearson and deviance procedure are .422 and 1, that is > .05, so the multinomial logistic regression model with nine predictor variables indicated a good fit (see Table 5).
Table 5
Goodness of fit with nine predictor variables
χ2 | df | p | |
---|---|---|---|
Pearson | 2449.03 | 2436 | .422 |
Deviance | 1381.48 | 2436 | 1.00 |
The Nagelkerke pseudo R2 in a multinomial regression analysis should be treated as a measure of effect size, similar to how R2 is treated in a standard multiple linear regression model (e.g., coefficient of the determination). In our analysis the Nagelkerke R2 was .16, suggesting that 16% of the variability is explained by this set of variables used in the model.
The classification table (see Table 6) provides the information on how well the model is able to predict the correct category for each case. For the overall cases, the model has correctly classified 69.0% of the respondents. There is one group that has very high levels of accurate prediction (97% for No Victim–No Perpetrator). A lower value was obtained for Victim Only (12.4%), Victim–Perpetrator (4.3%) and Perpetrator Only (3.2%).
Table 6
Classification table
The benchmark that is used to characterize a multinomial regression model as useful is a 25.0% improvement over the rate of accuracy achievable by chance alone. The proportional by chance accuracy rate was computed by calculating the proportion of cases for each group based on the number of cases in each group and then squaring and summing the proportion of cases in each group (0.682 + 0.202 + 0.092 + 0.042 = 0.511). The proportional by chance accuracy criterion (more than 25% than the chance) however was (1.25 × 51.1%) 63.9%. As previously stated, the classification accuracy rate was 69.0% (see Table 6), which was greater than the proportional by chance accuracy criterion of 63.9%. This suggests that that the model improves on the proportion by chance accuracy rate more than 25.0%, so that the criterion for classification accuracy is satisfied and the model is useful.
Table 7
Multinomial logistic regression analysis
Variables included in the model that predict being a victim of cyberbullying (but not a perpetrator) were age (OR = 0.83, 95% CI [0.69, 0.99]), prosocial behaviours (OR = 1.54, 95% CI [1.11, 2.14]), peer social support (OR = 0.55, 95% CI [0.43, 0.71]) and family social support (OR = 0.61, 95% CI [0.71, 1.22]). Being older and, more importantly, having greater social support (from both family and peers) reduce the probability of being a victim of cyberbullying (compared to people who do not experience or engage in cyberbullying). On the other hand, a higher level of prosocial behaviours increases this probability.
A higher level of social support from both peers (OR = 0.63, 95% CI [0.44, 0.91]) and family (OR = 0.56, 95% CI [0.42, 0.75]) decreases the probability of being a person who engages in or experiences cyberbul-lying (compared to persons who are not victims or perpetrators). The probability of being a victim but not a perpetrator also increases with the assertiveness level (OR = 1.68, 95% CI [1.17, 2.42]).
Only two variables in the model predict being a perpetrator (but not a victim): prosocial behaviours (OR = 0.57, 95% CI [0.33, 0.99]) and assertiveness (OR = 2.42, 95% CI [1.40, 4.20]). Greater intensity of prosocial behaviours reduces the likelihood of engaging in cyberbullying (but not being a victim of it); on the other hand, a higher level of assertiveness increases the probability of it.
DISCUSSION
The study was aimed at examining the prevalence of cyberbullying in Polish adolescents, covering four groups (bullies only; victims only; bully and victims; no bullies and no victims), as well as at relationships between selected factors, such as cognitive empathy, assertiveness, cooperation, pro-social behaviour, social support, and cyberbullying.
Dividing respondents into four groups revealed that Polish pupils do not experience cyberbullying very often since the majority of respondents did not report having had such experiences in the last six months. However, a significant group still experienced cyberbullying regularly (28.1%). Generally, the prevalence rates found in our study are comparable with other reports (Kowalski et al., 2014; Len-hart et al., 2010; Park et al., 2021; Patchin & Hinduja, 2016), while there are still discrepancies among the rates reported in different studies. We should note that such discrepancies could be partly attributed to differences in definitions and measures of cyberbul-lying. It is worth noting here that harassment was reported as the most frequently experienced form of cyberbullying. The majority of respondents who reported having experienced cyberbullying were victims only; fewer respondents were both bullies and victims.
In contrast, engaging in cyberbullying appeared to be even less common: it was reported by 12.3% of Polish pupils. The group that engaged in cyberbullying but did not experience it was even smaller: 3.8%. Generally speaking, we may conclude that cyberbullying among Polish students, while not common, occurs to such an extent that it requires special attention.
Family and peer social support appeared to be the strongest protective factor against experiencing cyberbullying, which confirms the widely accepted thesis of the beneficial function of social support. Strong social support enhances positive mood and general well-being. Recently, similar results were reported by Shaheen et al. (2019). Our results also indicated that being more prosocial makes a person less likely to engage in cyberbullying but more likely to experience it. This is understandable because prosocial behaviours are by nature the opposite of aggression. It is worth noting here that the protective role of pro-social behaviours was not addressed directly in other studies on cyberbullying. It is more difficult to explain why being prosocial makes an individual more likely to experience cyberbullying. It is possible that presenting prosocial behaviour provokes perpetrators in some way. Another explanation could be that prosocial behaviour goes against the peer acceptance of bullying (Walrave & Heirman, 2011).
The revealed role of assertiveness as a characteristic that makes a person more likely to engage in cyberbullying (bullies only and bully-victims) may be explained by assuming that respondents who were assertive were more likely to respond more strongly to harm experienced from peers by engaging in cyberbullying. While some studies have indicated that assertiveness may be a factor that protects against experiencing cyberbullying (Avsar & Ayas Alkaya, 2017; Rodriguez-Hidalgo et al., 2019), its role as a factor that protects against engaging in cyberbullying is less obvious (Avşar & Ayaz Alkaya, 2017). We may suppose that assertive adolescents appear to their peers as being stronger and thus provoking less aggression. The idea of imbalance of power in cyberbullying (Patchin & Hinduja, 2016) could be applied here.
The results indicating that cognitive empathy was not a significant protective factor are more difficult to explain. It is possible that its influence as a protective factor was not as strong as the influence of other factors, i.e. social support, and thus it appeared to be non-significant when analysed together. The influence of cognitive empathy could also be weakened because of some characteristics of cyberbullying, e.g. the fact that the victim’s reactions are not always directly visible or can be filtered by the computer screen.
There are some limitations of the reported study that should be mentioned. The cross- sectional design makes it difficult to draw conclusions concerning causality. It is also possible that due to social desirability some behaviours were not reported fully by respondents because they gave answers in the presence of the interviewer. Moreover, while the sample was relatively large it was not completely representative. Another limitation was the small sample size of one of the categories of respondents (perpetrator only; n = 31), which may reduce the power of statistical analyses. To estimate statistical power, we used post-hoc Monte Carlo simulation with a sample size of 822 and the results of our study (multinomial regression) as population values with 1000 replications. Power is evaluated using the proportion of replications for which the null hypothesis that a regression parameter is equal to zero is rejected at the .05 level, that is, the probability of rejecting the null hypothesis when it is false. Following Cohen (1988), statistical power estimates were to be 0.80 or above. For the perpetrator only group, post hoc power analyses indicated that only for two variables (pro-social behaviour – PSB and assertiveness – AS) was statistical power greater than 0.8 (for PSB it was 0.82, for AS 0.90). None of the other regression coefficients exceeded the value 0.8 (the maximum value was 0.30). Thus, the current study was powered to detect the observed effects. Nonetheless, replication of the current results with a larger sample size would provide greater confidence in the findings.
Further studies should examine the role of assertiveness more thoroughly, especially its role as a factor that protects against engaging in cyberbul-lying. Identifying individuals who are assertive but not aggressive could be helpful. It would be also recommended to examine the role of the characteristics examined in our study that, in light of the obtained results, appeared to be neither significant risk nor protective factors, namely cognitive empathy, cooperation and gender.
The results of our study can serve as guidance for preventive interventions against cyberbullying based on empirical evidence concerning the psychosocial risk factors of cyberbullying behaviour. Training focused on promoting prosocial behaviours and assertiveness as well as programmes enhancing social support in families and in schools could help in limiting cyberbullying. Initiatives to tackle cyberbullying should involve entire school communities: training programmes that focus on presenting the negative consequences of cyberbullying and on promoting protective behaviours and attitudes should include both pupils and teachers. In order to reduce the risk factors and the negative consequences of bullying, it is also necessary to implement preventive measures at the primary level, which means working with families. Besides helping to understand the nature and consequences of bullying, prevention should be based on teaching certain behaviours and ways of thinking. Both parents and children should be trained in recognizing and managing their emotions as well as developing concern for others. Moreover, children exposed to cyberbullying should be taught how to be assertive in defence against bullying.